Artificial Intelligence (AI) is rapidly reshaping transportation by improving safety, efficiency, and sustainability across various applications. From real-time traffic monitoring to predictive infrastructure maintenance, AI is becoming a critical tool for advancing transportation systems in New Jersey and nationwide. This article covers the use of AI in transportation research and implementation, with examples from the 2024 NJDOT Research Showcase, New Jersey and other state DOTs.
AI on Display at the 2024 Research Showcase
NJDOT held its 2024 Research Showcase on October 23, highlighting innovative transportation research and its implementation throughout New Jersey. During the morning panel discussion, Giri Venkiteela, Innovation Officer in NJDOT’s Bureau of Research, Innovation & Information Transfer, stated that Artificial Intelligence (AI) held significant promise for producing economic and environmental advancements in transportation due to its real-time predictive capabilities and proposed that NJDOT adopt protocols that can adapt to the pace of AI. Similar insights were heard throughout the showcase, where AI emerged as a central theme across numerous presentations and discussions.
AI, encompassing subcategories like Machine Learning (ML) and Artificial Neural Networks (ANN), allows researchers to analyze and model large data sets in real-time, saving significant labor hours and producing efficient, immediate results. Throughout the showcase, various projects ranging from enhancing pedestrian safety to predicting natural disasters utilized AI-based models.
Deep Patel received the 2024 Outstanding University Student in Transportation Research. As part of a research team at Rowan University, Patel deployed the AI model, YOLO-v5, to analyze video data from multiple New Jersey intersections, providing information on pedestrian volumes, traffic volumes, and the rate of vehicles running red lights, among other variables. The team then ranked intersection safety using the metrics analyzed by the AI model.
Patel’s research exemplifies the growing trend of integrating AI methods into traffic safety analyses, which continued into several presentations given in the afternoon Safety Breakout Sessions. Here, Rutgers professor Meiyin Liu presented her method for estimating real-time traffic flow through a combination of Unmanned Aerial Systems (UAS) and deep learning algorithms. A computer-mounted UAS would be used to record video data of a highway, which then gets transmitted to the YOLO-v5 computer vision AI that detects vehicle volume and estimates speed. This data collection method facilitates a real-time traffic flow analysis across a comprehensive geographic coverage that could enhance traffic performance and crash risk prediction. Afterward, Branislav Dimitrijevic, a member of an NJIT research team, showcased an AI-driven project that utilized LiDAR technology and YOLO-v5 computer vision to activate a Rectangular Rapid Flashing Beacon (RRFB) when pedestrians approached crosswalks, enhancing road safety.
Multiple posters featured at the Research Showcase contained elements of AI, including a poster titled “Integrating AI to Mitigate Climate Change in Transportation Infrastructure” made by Indira Prasad and “Artificial Intelligence Aided Railroad-Grade Crossing Vehicular Stop on Track Detection and Case Studies” highlighted by researchers at Rutgers’ CAIT.
AI’s critical role in the maintenance and preservation of infrastructure was also evident in the afternoon’s Sustainability Breakout Sessions. Indira Prasad, a Stevens Institute of Technology graduate student, conducted a review of future innovations in sustainable and resilient infrastructure. Prasad explained how AI’s pattern recognition capabilities could be used to analyze large data pools and help forecast natural disasters, enabling a rapid response to augment existing infrastructure. Surya Teja Swarna, a Rowan University postdoctoral researcher, demonstrated an innovative approach where state DOTs could use mobile phones mounted on vehicles to record roadway surface deformations, which then would be analyzed in real-time by an AI computer vision software, drastically reducing the time and costs required for road condition assessments.
Deployment of AI in Programs and Project Implementation
In addition to research from academic institutions, State DOTs and various other state, local and public transportation organizations have started to deploy AI-based methods and tools on various programs and projects.
Peter Jin, a Rutgers professor, received the 2024 NJDOT Research Implementation Award for his role in the New Brunswick Innovation Hub Smart Mobility Testing Ground (Data City SMTG). The project, created in partnership with NJDOT, the City of New Brunswick, and Middlesex County, functioned as a living laboratory for transportation data collection, containing Self-Driving Grade LiDAR sensors and computing devices across a 2.4-mile multi-modal corridor. Private and public sectors can use the data to enhance their advanced driving systems, automated vehicle models, and other AI-based projects.
Additionally, NJDOT has established a program integrating unmanned aerial systems (UAS) into its transportation operations. UASs provide high-quality survey and data mapping information, which, when paired with AI-based technologies, can be analyzed in real time to document roadway characteristics or conduct damage assessments for natural disasters. Meiyin Liu’s real-time traffic flow assessment research is one example of how UAS can be paired with AI.
The use of AI for railroad-grade crossing detection has been demonstrated on several projects in recent years. NJ TRANSIT, the statewide transit agency, recently received a $1.6 million grant from USDOT to implement a railroad-grade crossing detection system. The system, developed in partnership with CAIT researchers, will be deployed at 50 grade crossings and aboard five light rail vehicles throughout the state. The railroad-grade crossing detection system features multiple cameras on grade crossings and light rail vehicles to record data for an AI computer vision model that monitors and analyzes grade crossing behavior such as near-miss incidents.
For a project recently completed with the Federal Railroad Administration, CAIT researchers examined “stopped-on-track” incidents, which are a leading cause of grade-crossing accidents. During the poster session at the 2024 NJDOT Research Showcase, CAIT’s researchers highlighted a detection system for identifying stopped-on-track incidents and case study examples of how the critical locations can be addressed through design or other interventions. They found that targeted intervention using the AI detection system could reduce stopped-on-track incidents by up to 86 percent.
Other State DOTs have also started to implement AI-based programs. The Georgia Department of Transportation, in partnership with Georgia Tech, completed a survey of 22,000 road signs around potentially dangerous road curves using AI and vehicle-mounted mobile phone cameras to improve safety at road curves. The Texas Department of Transportation (TxDOT) assessed pavement conditions using LiDAR and AI. TxDOT’s project shares similarities with the research presented by Surya Teja Swarna, but it utilized LiDAR instead of a mobile phone camera.
In 2022, the Nevada Department of Transportation partnered with the Nevada Highway Patrol, the Regional Transportation Commission of Southern Nevada, and a private technology company to launch an AI-based platform that facilitated the reporting of real-time crash locations. A study on this project found that the AI platform uncovered 20 percent more crashes than previously reported and reduced emergency response time by nine to ten minutes on average while eliminating the need to dial for help.
Recent National Research
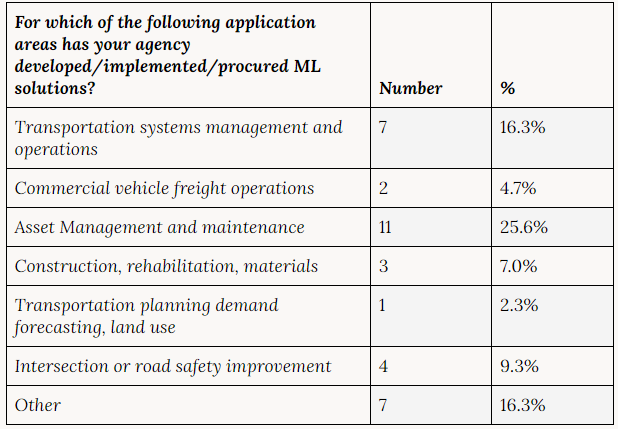
The National Cooperative Highway Research Program (NCHRP) published a 2024 research report, Implementing and Leveraging Machine Learning at State Departments of Transportation, that identifies trends in AI transportation research and implementation with a specific focus on machine learning and creates a roadmap for future implementation. The researchers surveyed State DOTs on plans regarding AI, reported case studies of ML implementation by State DOTs, and listed strategies to help DOTs facilitate further inclusion of AI solutions.
The survey of the state DOT officials covered various topics, including the transportation agency’s familiarity with AI methods and tools, types of methods and applications utilized, and challenges in implementation. Among the challenges to implementation, DOT officials noted a lack of public trust, insufficient data collection and storage infrastructure, and, most commonly, scarce labor with knowledge of AI. Most computer and data scientists choose to work in the private sector, and it can be difficult to recruit them to a transportation agency.
The NCHRP report also included multiple case studies from state DOTs such as Nebraska, California, and Iowa, documenting the experiences of these agencies in developing and implementing ML programs.
- Nebraska DOT (NDOT) used a computer vision Convolutional Neural Network (CNN) algorithm to detect and analyze guardrail quality. NDOT recorded 1.5 million images of guardrail data and used AI to save time and money compared to the manual detection alternative. Among the challenges, NDOT observed that their agency did not have the necessary infrastructure to process large volumes of data and lacked in-house ML expertise. The agency solved the former issue by using a private vendor to process the data and the latter by collaborating with consultants from the University of Nebraska. The algorithm achieved accuracies of 97 percent for guardrail detection and 85 percent for their classification into three types.
- The California Department of Transportation (Caltrans) has leveraged AI/ML applications across various projects and partnered with numerous tech companies, including Google. One area of emphasis for Caltrans has been workforce capacity development. While most staff do not have experience with AI-based data analytics, they do have experience with GIS. Caltrans has worked with GIS tool developers to incorporate ML functionalities into the basic user interface of GIS programs, making it more intuitive for their workforce.
- Iowa State University, funded by the Iowa Department of Transportation, developed a real-time ML tool to monitor highway performance, enabling a rapid response to traffic congestion. The researchers identified the need for high-performance computing as a significant challenge preventing large-scale implementation. Mass deployment of the tools used in the research study would require a considerable expense, partially due to the stipulation that the code be at least 99 percent reliable.
For more information on the application and implementation of AI by transportation agencies, the National Academies of Sciences, in collaboration with the NCHRP, published two additional reports in 2024. One, Artificial Intelligence Opportunities for State and Local DOTs: A Research Roadmap, utilizes machine learning methods to analyze research trends in AI and how State DOTs can implement the research. The other, Implementing Machine Learning at State Departments of Transportation: A Guide, serves as a complementary document to the NCHRP report on implementing and leveraging machine learning.
On a national level, USDOT published its Advancing Governance, Innovation, and Risk Management for Agency Use of Artificial Intelligence compliance plan in September 2024. USDOT has taken several measures to advance the implementation of AI, including forming an AI Governance Board chaired by the Deputy Secretary and vice-chaired by a new Chief Artificial Intelligence Officer (CAIO), creating an AI Accelerator Roadmap, and providing funds for AI research and implementation.
Lastly, the American Association of State Highway and Transportation Officials (AASHTO) hosted a knowledge session examining the role of AI in transportation in April 2024. Practitioners on the panel highlighted the potential of AI in eliminating the dangerous aspects of data collection and allowing for proactive solutions rather than reactively responding to crashes or injuries. The panelist discussion touched upon the importance of building trust in a period of rapid AI development, noting the critical role that academic researchers can play as partners with state DOTs to advance and develop the AI technology in ways beneficial for traffic safety and workforce safety, among other topics.
TRID Database
Artificial Intelligence-based research can be found via TRB’s TRID database. The following are some relevant articles published on recent New Jersey transportation research in AI.
- Bagheri, M., B. Bartin, and K. Ozbay. (2023). Implementing Artificial Neural Network-Based Gap Acceptance Models in the Simulation Model of a Traffic Circle in SUMO. Transportation Research Record: Journal of the Transportation Research Board, Vol. 2677. https://trid.trb.org/View/2166547
- Hasan, A.S., M. Jalayer, S. Das and M. Bin Kabir. (2024). Application of machine learning models and SHAP to examine crashes involving young drivers in New Jersey. International Journal of Transportation Science and Technology, Vol. 14. https://trid.trb.org/View/2162338
- Hasan, A.S., M. Jalayer, S. Das and M. Bin Kabir. (2023). Severity model of work zone crashes in New Jersey using machine learning models. Journal of Transportation Safety & Security, Vol. 15. https://trid.trb.org/View/2190127
- Najafi, A., Z. Amir, B. Salman, P. Sanaei, E. Lojano-Quispe, A. Maher, and R. Schaefer. (2024). A Digital Twin Framework for Bridges. ASCE International Conference on Computing in Civil Engineering 2023, American Society of Civil Engineers, pp 433-441. https://trid.trb.org/view/2329319
- Nayeem, M., A. Hasan, M. Jalayer. (2023). Investigation of Young Pedestrian Crashes in School Districts of New Jersey Using Machine Learning Models. International Conference on Transportation and Development 2023, American Society of Civil Engineers. https://trid.trb.org/View/2196775
- Patel, D., P. Hosseini, and M. Jalayer. (2024). A framework for proactive safety evaluation of intersection using surrogate safety measures and non-compliance behavior. Accident Analysis & Prevention, Vol. 192. https://trid.trb.org/View/2242428
- Zaman, A., Z. Huang, W. Li, H. Qin, D. Kang, and X. Liu. (2023). Artificial Intelligence-Aided Grade Crossing Safety Violation Detection Methodology and a Case Study in New Jersey. Transportation Research Record: Journal of the Transportation Research Board, Vol. 2677. https://trid.trb.org/VCiew/2169797
- Zaman, A., Z. Huang, W. Li, H. Qin, D. Kang, and X. Liu. (2024). Development of Railroad Trespassing Database Using Artificial Intelligence. Rutgers University, New Brunswick, Federal Railroad Administration, 80p. https://trid.trb.org/view/2341095
Additional Resources
- Federal Highway Administration. (2021). Implementation of Artificial Intelligence to Improve Weather Maintenance. Washington DC. https://www.fhwa.dot.gov/publications/research/ear/21090/21090.pdf
- Federal Transit Administration. (2023). Utilizing Artificial Intelligence with Vision-Based Systems for Monitoring Trespassing – Best Practices. Center for Urban Transportation Research, University of South Florida. FTA Report No. 0256. https://www.transit.dot.gov/sites/fta.dot.gov/files/2023-10/FTA-Report-No-0256.pdf
- National Academies of Sciences, Engineering, and Medicine. (2024). AI Applications for Automatic Pavement Condition Evaluation. Washington, DC: The National Academies Press. https://nap.nationalacademies.org/catalog/27993/ai-applications-for-automatic-pavement-condition-evaluation
- National Academies of Sciences, Engineering, and Medicine. (2024). Artificial Intelligence Opportunities for State and Local DOTs: A Research Roadmap. Washington DC: The National Academies Press. https://nap.nationalacademies.org/catalog/27865/artificial-intelligence-opportunities-for-state-and-local-dots-a-research
- National Academies of Sciences, Engineering, and Medicine. (2024). Implementing Machine Learning at State Departments of Transportation: A Guide. Washington, DC: The National Academies Press. https://nap.nationalacademies.org/catalog/27880/implementing-machine-learning-at-state-departments-of-transportation-a-guide
- National Cooperative Highway Research Program. (2024). Implementing and Leveraging Machine Learning at State Departments of Transportation. Washington, DC: The National Academies of Sciences and Transportation Research Board. https://nap.nationalacademies.org/catalog/27902/implementing-and-leveraging-machine-learning-at-state-departments-of-transportation
- New Jersey AI Task Force. (2024). Report to the Governor on Artificial Intelligence. The State of New Jersey. https://www.nj.gov/governor/docs/Final-2024-NJ-AI-Task-force-Report-to-Governor.pdf
- Rail Safety, I. D. E. A. (2024). An Artificial Intelligence Aided Forward-Facing Camera Video Data Analytics System for Rail Safety. The National Academies of Sciences and Transportation Research Board. https://www.trb.org/Main/Blurbs/183277.aspx
- Transportation Research Board. (2024). TRB Snap Search: Artificial Intelligence. https://onlinepubs.trb.org/onlinepubs/snap/ArtificialIntelligence.pdf
- United States Department of Transportation. (2024). US Department of Transportation (DOT) Compliance Plan for Memorandum M-24-10 (September 2024). https://www.transportation.gov/sites/dot.gov/files/2024-09/USDOT_Compliance_Plan-for-OMB_Memorandum_M-24-10_%28September_2024%29.pdf