Traffic safety and mobility, two critical areas in transportation engineering, both require the collection and analysis of large data sets to produce proactive and comprehensive solutions. Transportation engineers have started to increasingly focus on using innovative technologies to efficiently and effectively process this data.
We had the opportunity to speak with Dr. Deep Patel, a former Ph.D. candidate and research fellow at Rowan University, whose work is at the forefront of this mission. Recently, Patel received the NJDOT Outstanding University Student Research Award for his contributions to transportation research. In this interview, Patel shares insights from his research journey, including his work on the Real-Time Traffic Signal Performance Measurement Study and the development and implementation of machine learning tools to predict high-risk intersections. His dedication to improving traffic operations and safety, along with his new industry role as a Traffic Safety and Mobility Specialist, highlights the significant impact of combining academic research with practical industry applications.
Q. Could you tell us about your educational and research experience and how you became a PhD candidate and research fellow at Rowan University?
A. First of all, thank you for your time and for considering me for the opportunity to be interviewed about my recent NJDOT award. I would also like to thank the NJDOT review committee members and my Ph.D. advisor Dr. Mohammad Jalayer, who supported me in receiving this award.
I started my master’s study in 2018 as a civil engineering student without a research focus. Then, during my first semester, I took a course called Transportation Engineering with Dr. Mohammad Jalayer. When he sought traffic counting assistance for a traffic analysis project, I eagerly joined him, becoming his first research student.
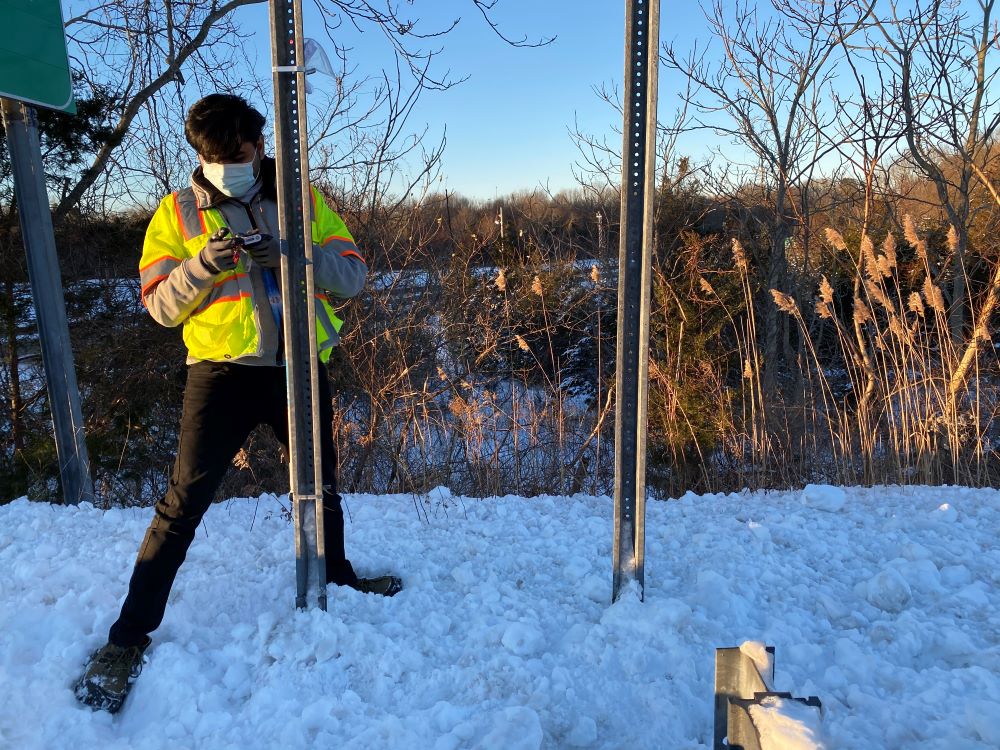
Through that experience, I started thinking about what could streamline the traffic counting process and the various uses for the data we collected. I went on to work on several research projects with Dr. Jalayer, both funded and non-funded, where we had frequent discussions, and I would present my ideas to him. Eventually, he asked me to join him as a researcher and to continue my master’s work with a research focus, which I did for two years. When he suggested I continue my studies to earn a Ph.D., I was initially surprised, but I decided to go for it since I had a lot of ideas for future research projects.
At the end of my master’s study, I began Phase One work for a Real-Time Traffic System Performance Measure Study led by Dr. Peter Jin, Dr. Thomas Brennan, and Dr. Jalayer. This project connected me with a team from Rutgers, TCNJ, and a few professionals from NJDOT and other industry folks. I represented Rowan’s end for this project, where our focus was on understanding the safety aspects including safety parameters and performance and how we could assist NJDOT transform this new technology to help save lives. For the first phase of the project, we worked on understanding the traffic signal system performance measures, and what had been adopted by other DOTs. My experience on this project drove me to pursue more research and to expand my knowledge in traffic safety.
Q. You worked on Phase One through Three of this Real-Time Traffic Signal Performance Measurement Study. What part of this project interested you the most?
A. My main takeaway from this project focused on learning more about how the transportation industry looks towards the research outputs and outcomes from the university teams. It is very interesting to understand how university-based research is being adapted for industry acceptance. Additionally, I learned what problem-solving features the industry looks for from the research component.
From a technical aspect, I learned how New Jersey signals can be enhanced and how we can optimize the performance of these signals and achieve cost savings. Let’s say you have a scenario where there is no vehicle at an intersection; how can we provide recommendations to change the signal to a red light and give the other side of the intersection a green light? So, we gathered several components in terms of mobility, safety, and economic parameters from the study that can help enhance our traffic signals in New Jersey, sharing this information with the NJDOT team.
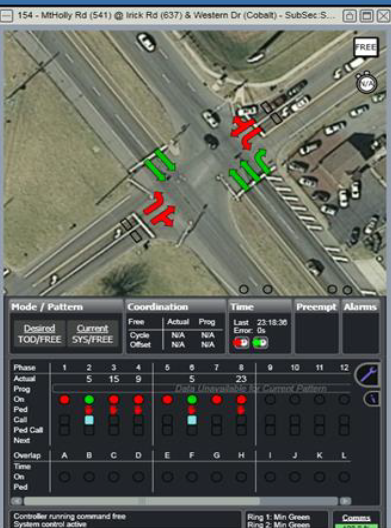
Q. How did you see your role on the research project develop as you moved from the earlier phases to the latest phase?
A. In the first phase, we completed a comprehensive literature review to understand what is happening across the nation, which systems are being adapted, what are the best systems for providing traffic signal safety performance measures, and what are the kind of performance measures that can be adapted in an industry setting. In Phase Two, the team focused on developing mechanisms and performance measures aligned with NJDOT’s existing data, including deploying the Automated Traffic Signal Performance Measures (ATSPM) system to enhance traffic signal monitoring and optimization. To guide these efforts, an adaptability checklist was created to benchmark practices from other states and identify strategies that could be adapted to benefit NJDOT’s operations. Building on this foundation, Phase Three advanced to the demonstration and application of dashboards and performance measures, providing actionable recommendations to NJDOT on enhancing mobility and safety across various regions and corridors. These efforts aimed to save time and lives, while the integration of connected vehicle (CV) technologies remains a key focus for future work, ensuring NJDOT’s leadership in traffic management innovation.
Q. What were the specific corridors that you worked on?
A. We started with seven/eight intersections on U.S. 1. Then, we explored the whole corridor of U.S. 1 as part of Phase Three, and we also brought in Route 18, Route 130, and other intersections during this phase.
Q. Did you discover any particular surprising or noteworthy findings from this research?
A. This was a long project, extending from 2019-2024. As a result, each year we discovered new findings, and new components were often added to the project. For example, we added a CV systems component as part of the Phase Two and Phase Three projects to start planning for the future and understand what kind of data could be received and sent from CV technologies. The main benefit from this project is that it not only established current problem-solving measures but also looked into the future, helping to better understand what’s coming and how we can best face anticipated challenges that we need to start integrating at this moment. I find the combination of the present and future integration of systems and technologies interesting and important from the findings.
Q. What kind of impact do you think you and your research will have on NJDOT traffic operations and traffic safety, especially with your role now working in the industry?
A. With my previous experience as part of a university-led research team and now as a Traffic Safety and Specialist in the private sector, I am better positioned to facilitate the efficient and effective implementation of research findings. A key factor enabling this transition is that Kelly McVeigh, who supervised the original research project, also oversees the current work that our firm is doing for NJDOT. Being on the industry side allows me to introduce and operationalize new ideas more rapidly, compared to the academic research side. This streamlined approach ensures that innovative performance measures can be deployed more quickly, and even a small modification has the potential to save lives, underscoring the value of this work.
Q. Moving to a different topic, your research frequently incorporates Machine Learning (ML) and Artificial Intelligence (AI) aspects. In your experience, what benefits does AI contribute to transportation research?
A. Over the past few years, AI and ML have undergone drastic modifications and growing levels of industry acceptance. Additionally, in research outcomes, AI and ML have played a key role in enhancing and providing new methodologies and new ways of problem-solving. As an engineer, the first thing we have to do is understand how we can solve an existing problem, and how fast, effectively, and efficiently we can do it.
Transportation is now highly reliant on big data and intensive analysis, so AI and ML back up the processing of this data, coming up with meaningful outcomes and enhancing solution measures much quickly than previous methods. In 2012 or 2013, a standard engineer would need to sit down to do a traffic study and go through manual counting, then process the data, then come up with solutions, which takes much longer to solve a problem. The problem may even change during the months-long process of developing a solution.
In traffic safety, we cannot wait for the four to five months it could take to solve a problem due to the pressing safety implications of doing so. Thus, we must start implementing countermeasures swiftly, and AI and ML components help us to quickly process data with more effective and efficient results.
During my early days as a student researcher, I would stand on the roadside, manually counting the vehicles and pedestrians to collect data for traffic studies. However. during my doctoral research, I developed my AI-driven tools that utilize advanced video systems for detection and analysis. This proactive approach enables the identification of intersections prone to high-crash scenarios well before crashes occur, allowing for timely interventions. By integrating AI and ML, my research introduced innovative methodologies for crash prediction and prevention, showcasing the feasibility of data-driven solutions to enhance roadway safety.
There is a certain chaos in human beings’ lives and surroundings that requires transportation to be a multidisciplinary field, which includes human-focused aspects. For some parts, AI is definitely required, but with other parts, we need to go through different approaches.
Q. Do you think that because of AI’s data collection and analysis possibilities, almost all engineers in the near future will need to start incorporating AI into their research?
A. It really depends. For our part of traffic engineering, very specifically, I would say yes, it would be one of the major requirements that an engineer would need to adopt. But if I was a traffic engineer working on policy or equity measures there might be some concerns related to data sharing or data privacy issues that might restrict them.
It depends on what side you are focusing on. When it comes to data collection, I would say AI incorporation is a must to collect and process data faster and more efficiently. But in terms of developing policies, rules, or statutes, there are certain psychological aspects that need to be in the thought process. Knowing human concerns and people’s approaches requires an emotional touch, which AI still lacks.
Transportation is a field connected with multiple disciplines; it touches on people’s emotions. For example, on a day when traffic does not work well when you’re returning home, you can get frustrated, and that frustration can end up in a fatal crash. There is a certain chaos in human beings’ lives and surroundings that requires transportation to be a multidisciplinary field, which includes human-focused aspects. For some parts, AI is definitely required, but with other parts, we need to go through different approaches.
Q. Congratulations on your recently approved dissertation. Could you give us some quick highlights of the research methods that went into producing your dissertation, “A Comprehensive ML and AI Framework for Intersection Safety”? What are the most important takeaways from your dissertation?
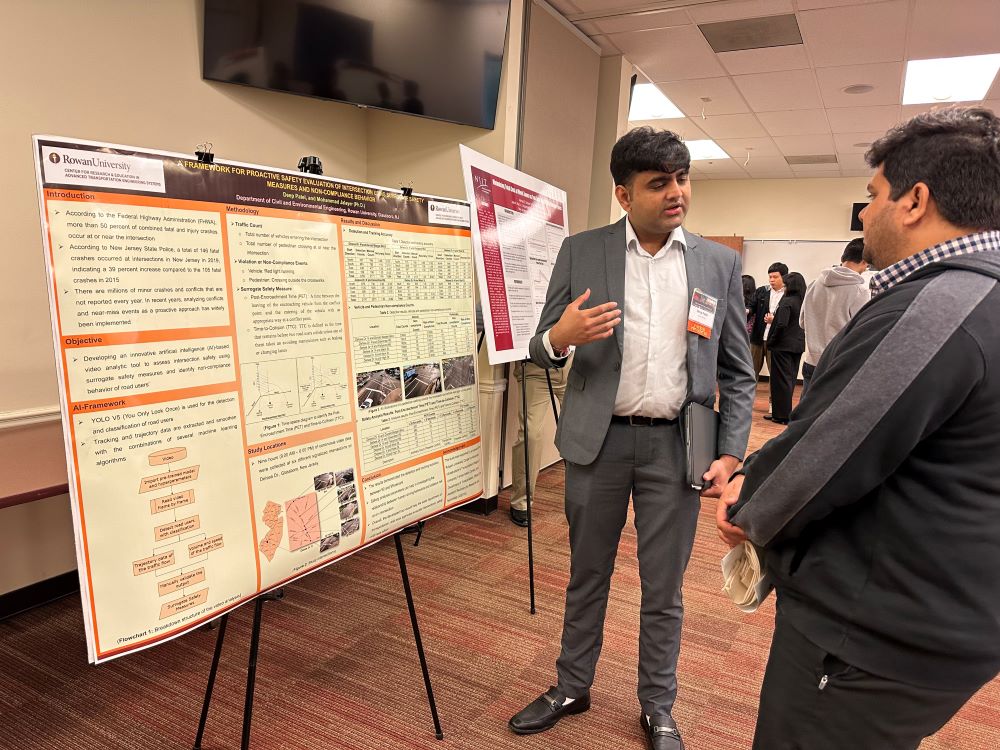
A. New Jersey is home to some of the most dangerous intersections in the United States, with four intersections ranked among the top 15 most dangerous, including the 1st, 2nd, and 3rd positions. Since 2019, there has been a trend of steadily increasing intersection-related crashes and correlated crashes within intersection boundaries. This prompted me to ask, “Why do we need to wait for crashes to happen to address the problem?”
To tackle this issue, I developed a proactive approach inspired by my work on the NJDOT research project. The approach focuses on analyzing near-miss incidents and traffic violations, using the concept of surrogate safety measures to identify potential risks before crashes occur. Surrogate safety measures help us detect near-miss events and violations, offering a predictive understanding of high-risk scenarios at intersections.
Using AI and ML, we developed tools that analyze vehicle and pedestrian trajectories in detail. These tools detect and classify conflicts, such as left-turn conflicts or yielding conflicts, enabling us to predict potential crash scenarios based on behavioral patterns at intersections. This proactive analysis allows us to recommend design changes and interventions before crashes occur.
Then, we explored the noncompliance component in a certain area, like red light violations or jaywalking. For instance, our analysis revealed that one in every four pedestrians does not use crosswalks. By integrating historical crash data, proactive trajectory analysis, and noncompliance trends, we developed a tool that ranks intersections based on multiple criteria. These include potential high-crash scenarios, contributing factors, and the economic impact of injury severity at specific locations.
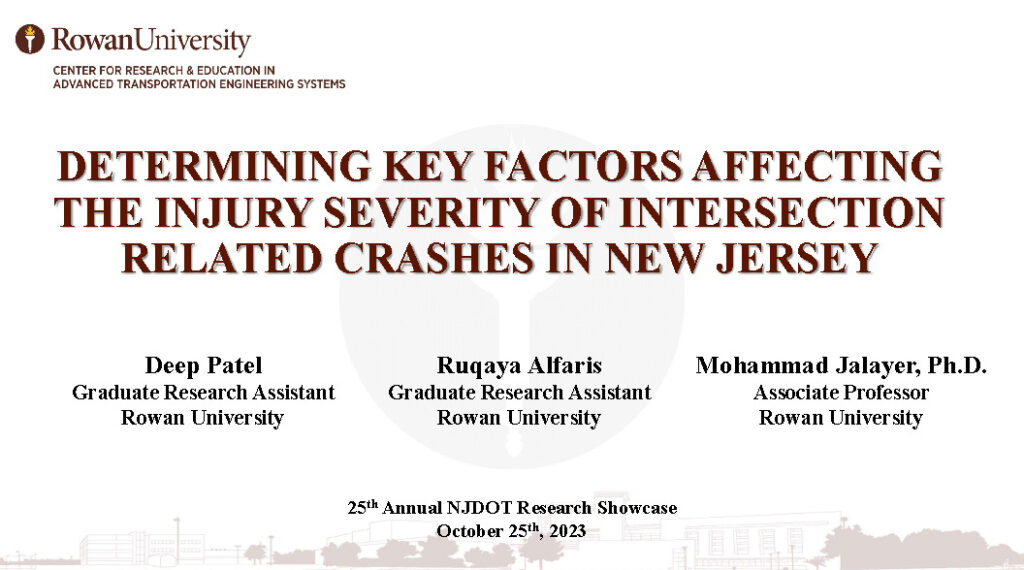
Additionally, the research explored how emerging technologies, such as connected and autonomous vehicles, could be adapted to enhance intersection safety. By conducting trajectory analyses, we assessed how data from these technologies could inform future safety measures and interventions.
Overall, my research focused on identifying key factors within intersection boundaries to reduce crashes, improve mobility, and do so in a cost-effective manner. This comprehensive approach combines proactive analysis, advanced technologies, and human behavior insights to deliver practical and impactful solutions for roadway safety.
Q. So this tool seems to be one of the most important takeaways. Is the tool ready for NJDOT use to identify potential high crash risk intersections? Is that the main intent of the tool?
A. Yes, exactly. The tool is ready but not yet publicly available. We tested it on several intersections. It is currently a proprietary tool of my professor and myself at Rowan University. Anyone interested in using the tool can connect with us, but it is not yet publicly available and certain permissions are required.
Q. Is NJDOT using it or can they use it?
A. No, the department is not using it because this was part of my recent defense. They are aware of the tool’s capabilities because it was part of an innovative showcase. The tool’s documentation has been published through the University Transportation Center (UTC). Hopefully, in the near future, it could be applied by NJDOT.
Q. Looking ahead, you have your new position in an industry role. Would you like to continue with this sort of focus on transportation research, or are you anticipating a different career direction?
A. With my new position as a Traffic Safety and Mobility Specialist, I will be focused on transportation research, conducting high-quality industry research where I would help develop safety and mobility performance measures on certain corridors designed to move traffic more effectively and enhance safety on the roadways. My work will also include industry deployment and understanding the agencies’ concerns regarding the challenges they face.
Looking ahead, I see my career direction as a blend of research and practical implementation, ensuring that innovative solutions are not just developed but also applied to make a real-world impact. Ultimately, if my work can contribute to saving even a single life, I will consider it a meaningful and worthwhile achievement.
Resources
Jin, P. J., Zhang, T., Brennan Jr, T. M., & Jalayer, M. (2019). Real-Time Signal Performance Measurement (RT-SPM) (No. FHWA NJ-2019-002). Retrieved at: https://www.njdottechtransfer.net/wp-content/uploads/2020/01/FHWA-NJ-2019-002.pdf
Jin, P. J., Zhang, T., Brennan Jr, T. M., & Jalayer, M. (2019). Real-Time Signal Performance Measurement Phase II. Retrieved at: https://www.njdottechtransfer.net/wp-content/uploads/2022/08/FHWA-NJ-2022-002-Volume-I-.pdf
Patel, D., P. Hosseini, and M. Jalayer. (2024). A framework for proactive safety evaluation of intersection using surrogate safety measures and non-compliance behavior. Accident Analysis & Prevention, Vol. 192. https://trid.trb.org/View/2242428
Patel, D. (2024). “A Comprehensive ML and AI Framework for Intersection Safety: Assessing Contributing Factors, Surrogate Safety Measures, Non-Compliance Behaviors, and Cost-Inclusive Methodology.” Theses and Dissertations. 3305. https://rdw.rowan.edu/etd/3305
For more information about the 26th annual NJDOT Research Showcase, visit: Recap: 26th Annual NJDOT Research Showcase